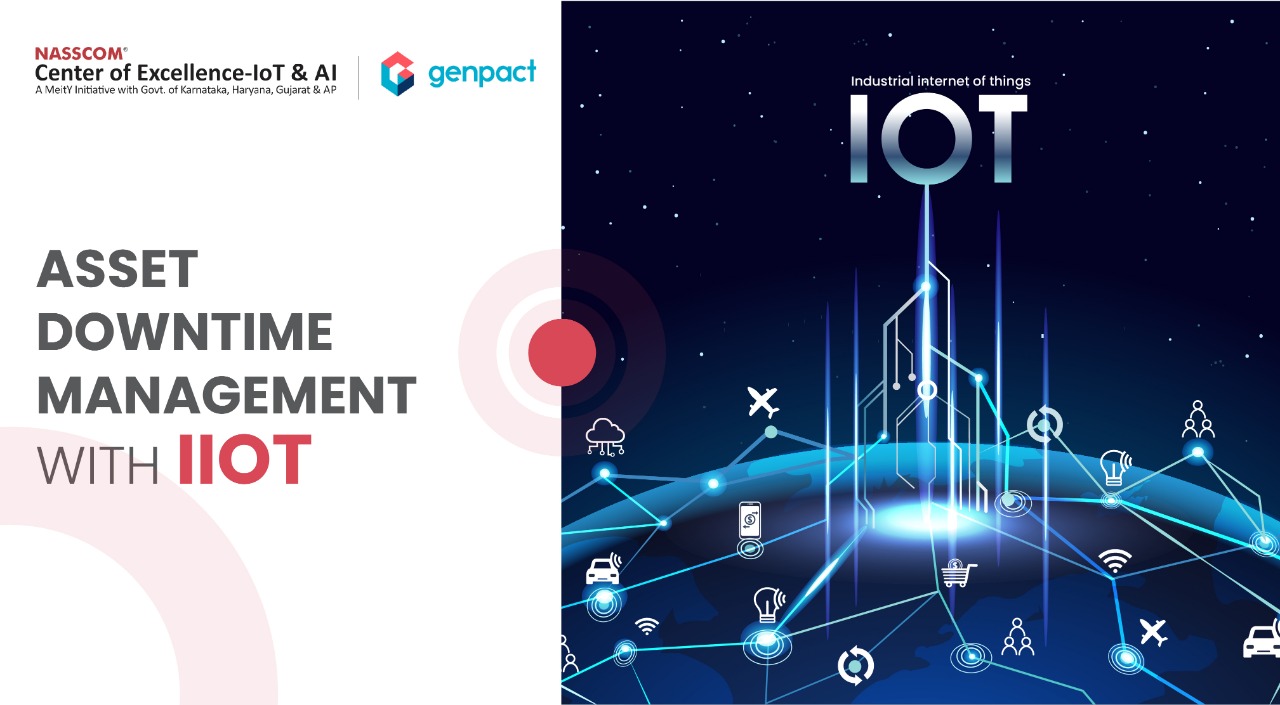
Genpact Supply Chain Practice
Heavy industrial equipment manufacturers (e.g., OEMs) that produce Industrial equipment products on the field such as aircraft engines, gas turbines, locomotives, etc., typically lose track of critical asset attributes, from a standpoint of operational and technical parameters, once they are sold to customers. These products often cost millions of dollars and are in operation over multiple decades.
As a result, there is a high probability of losing abilities to trace future equipment service maintenance opportunities accurately as well as associated aftermarket service revenues. Such circumstances also result in improper planning in terms of parts and repairs, field service technician deployment, and slot scheduling at the repair shop for maintenance activity execution. As a result, equipment downtime leaves high costs for OEM and dissatisfied customers due to long lead maintenance cycle time.
Achieving the identification of aftermarket service revenue by the traditional approach with just non-real-time data has been not very effective. This is predominantly because of challenges, such as disjointed accessibility to asset datasets, data processing time, the accuracy of the data, and lack of integration between maintenance policies, which result in improper execution of maintenance activity.
To address the above-mentioned issues, smart and proactive management of equipment downtime is essential which should focus on carrying out experimentation on data (real-time and non-real-time) from equipment with the aid of industrial internet (IIOT) technologies, harnessing algorithms and analytics to achieve the following:
Improve data quality for aftermarket service forecasting:
How? Traditional data sources have quality issues and have not been fully utilized for maintenance. Leveraging real-time sensor data from connected assets. Utilizing data extraction techniques from legacy systems and perform automated supervised learning-based quality checks to identify the source of quality degradation. Deploy machine learning (ML) driven data monitoring and purification techniques to improve data quality.
Increase the accuracy of aftermarket service revenue forecast:
How? Validate the current predictive forecasting approach, harness real-time/non-real-time data, and compare with different types of equipment downtime, the right usage of maintenance policies to improve the accuracy of the forecast.
Reduce aftermarket service lead time for asset maintenance:
How? Usage of derived accurate forecast to reduce the lead time for equipment maintenance by enabling the proactive availability of parts, repairs and field technicians at right time (which potentially can take many months today).
Market opportunity (Illustration)
According to the International Energy Agency (IEA), new investments of around $1 trillion will go into building natural gas-fired power plants over the next two decades.
In 2016 alone, aftermarket service maintenance revenue for gas turbines was $40 billion. The opportunity of ~50 years of turbine life and extended to other similar assets (such as aircraft engines, locomotives, etc.) are at least an order of magnitude higher.
(The split includes parts, repairs, and technician services, and excludes new sales).
This structured approach of harnessing real-time data with maintenance data alongside maintenance policies will lead to not only accurate asset downtime predictions but also proactive identification of aftermarket service revenue for heavy industrial equipment manufacturers.
Other positives of this approach are that it aids the effective maintenance execution by proactively planning for parts and repairs; reduces the part procurement cycle time; leads to more timely deployment of field service technicians, and supports proactive scheduling of the right time slot at maintenance/repair shops (in the instances where the on-field repairs are difficult to achieve).
Overall, the embedment of industrial internet of things (IIOT) technologies provides a greater advantage in equipment downtime management in a proactive manner as compared to the traditional reactive manner, with an optimal cost and with the highest execution efficiency resulting in increased customer satisfaction.
Economic outcomes
Industries that will be able to take advantage of such an approach include heavy industrial machinery (aircraft engines, locomotives, etc.), energy and utilities (gas turbines, wind farms, etc.), and mining (rigs, cranes, etc.), among others.
- For an industrial equipment manufacturer aftermarket service revenue enhancement due to maintenance opportunity identification
- On time reach to customers or channel partners in reducing the revenue opportunity leakage to competition
Societal outcomes
For OEM: Provides an extensive visibility on asset outage manageability to ensure the cost of an outage is under profitable threshold with highest customer satisfaction.
For customer: Lesser asset down time, increase throughput resulting in better customer satisfaction and Net Promoter Score (NPS).
For any further information\query you can write to us at Co-innovate@nasscom.in